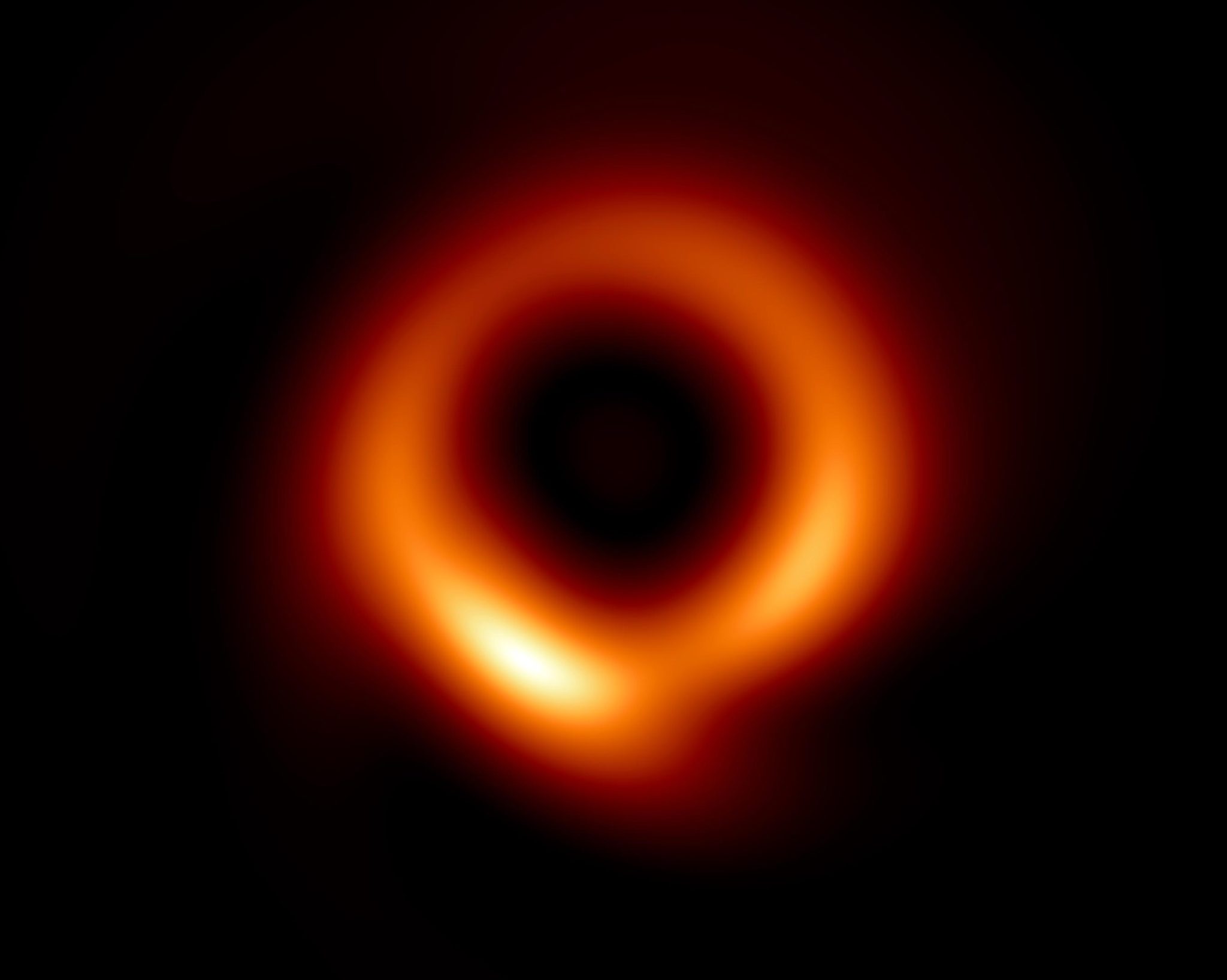
Nueva imagen del agujero negro supermasivo M87 generada por el algoritmo PRIMO utilizando datos EHT de 2017. Crédito: Medeiros et al. 2023
El aprendizaje automático reconstruye una nueva imagen a partir de los datos de EHT.
foto de m87[{” attribute=””>black hole has been enhanced using a machine learning technique called PRIMO, providing a more accurate representation and allowing for improved determinations of its mass and physical parameters.
The iconic image of the supermassive black hole at the center of M87—sometimes referred to as the “fuzzy, orange donut”—has gotten its first official makeover with the help of machine learning. The new image further exposes a central region that is larger and darker, surrounded by the bright accreting gas shaped like a “skinny donut.” The team used the data obtained by the Event Horizon Telescope (EHT) collaboration in 2017 and achieved, for the first time, the full resolution of the array.
In 2017, the EHT collaboration used a network of seven pre-existing telescopes around the world to gather data on M87, creating an “Earth-sized telescope.” However, since it is infeasible to cover the Earth’s entire surface with telescopes, gaps arise in the data—like missing pieces in a jigsaw puzzle.
M87 supermassive black hole originally imaged by the EHT collaboration in 2019 (left); and new image generated by the PRIMO algorithm using the same data set (right). Credit: Medeiros et al. 2023
“With our new machine learning technique, PRIMO, we were able to achieve the maximum resolution of the current array,” says lead author Lia Medeiros of the Institute for Advanced Study. “Since we cannot study black holes up close, the detail of an image plays a critical role in our ability to understand its behavior. The width of the ring in the image is now smaller by about a factor of two, which will be a powerful constraint for our theoretical models and tests of gravity.”
PRIMO, which stands for principal-component interferometric modeling, was developed by EHT members Lia Medeiros (Institute for Advanced Study), Dimitrios Psaltis (Georgia Tech), Tod Lauer (NOIRLab), and Feryal Özel (Georgia Tech). Their publication, “The Image of the M87 Black Hole Reconstructed with PRIMO,” was published today (April 13) in The Astrophysical Journal Letters.
“PRIMO is a new approach to the difficult task of constructing images from EHT observations,” said Lauer. “It provides a way to compensate for the missing information about the object being observed, which is required to generate the image that would have been seen using a single gigantic radio telescope the size of the Earth.”
Animation fades from M87 black hole image, first produced by the EHT collaboration in 2019, to the new image generated by the PRIMO algorithm using the same data set. Credit: Medeiros et al. 2023
PRIMO relies on dictionary learning, a branch of machine learning which enables computers to generate rules based on large sets of training material. For example, if a computer is fed a series of different banana images—with sufficient training—it may be able to determine if an unknown image is or is not a banana. Beyond this simple case, the versatility of machine learning has been demonstrated in numerous ways: from creating Renaissance-style works of art to completing the unfinished work of Beethoven. So how might machines help scientists to render a black hole image? The research team has answered this very question.
With PRIMO, computers analyzed over 30,000 high-fidelity simulated images of black holes accreting gas. The ensemble of simulations covered a wide range of models for how the black hole accretes matter, looking for common patterns in the structure of the images. The various patterns of structure were sorted by how commonly they occurred in the simulations, and were then blended to provide a highly accurate representation of the EHT observations, simultaneously providing a high fidelity estimate of the missing structure of the images. A paper pertaining to the algorithm itself was published in The Astrophysical Journal on February 3, 2023.
“We are using physics to fill in regions of missing data in a way that has never been done before by using machine learning,” added Medeiros. “This could have important implications for interferometry, which plays a role in fields from exo-planets to medicine.”
Descripción general de las simulaciones generadas para el conjunto de entrenamiento del algoritmo PRIMO. Crédito: Medeiros et al. 2023
El equipo confirmó que la imagen proporcionada recientemente es consistente con los datos de EHT y con las predicciones teóricas, incluido el anillo brillante de emisiones que se espera que resulte del gas caliente que cae en el agujero negro. Crear una imagen requiere asumir la forma adecuada de la información que falta, y PRIMO lo hizo basándose en el descubrimiento de 2019 de que el agujero negro M87 en gran detalle se veía tal como se predijo.
“Casi cuatro años después de revelar la primera imagen en escala horizontal de un agujero negro por EHT en 2019, hemos establecido otro hito, produciendo una imagen que utiliza la resolución completa de la matriz por primera vez”, afirmó Psaltis. “Las nuevas técnicas de aprendizaje automático que hemos desarrollado brindan una oportunidad de oro para que nuestro trabajo colectivo comprenda la física de los agujeros negros”.
La nueva imagen debería conducir a determinaciones más precisas de la masa del agujero negro M87 y los parámetros físicos que determinan su apariencia actual. Los datos también brindan una oportunidad para que los investigadores establezcan mayores restricciones en las alternativas del horizonte de eventos (basadas en un brillo central oscuro más bajo) y realicen pruebas de gravedad más sólidas (basadas en un tamaño de anillo más estrecho). PRIMO también se puede aplicar a observaciones adicionales de EHT, incluidas las de Sgr A*, el agujero negro central de nuestra región.[{” attribute=””>Milky Way galaxy.
M87 is a massive, relatively nearby, galaxy in the Virgo cluster of galaxies. Over a century ago, a mysterious jet of hot plasma was observed to emanate from its center. Beginning in the 1950s, the then new technique of radio astronomy showed the galaxy to have a compact bright radio source at its center. During the 1960s, M87 had been suspected to have a massive black hole at its center powering this activity. Measurements made from ground-based telescopes starting in the 1970s, and later the Hubble Space Telescope starting in the 1990s, provided strong support that M87 indeed harbored a black hole weighing several billion times the mass of the Sun based on observations of the high velocities of stars and gas orbiting its center. The 2017 EHT observations of M87 were obtained over several days from several different radio telescopes linked together at the same time to obtain the highest possible resolution. The now iconic “orange donut” picture of the M87 black hole, released in 2019, reflected the first attempt to produce an image from these observations.
“The 2019 image was just the beginning,” stated Medeiros. “If a picture is worth a thousand words, the data underlying that image have many more stories to tell. PRIMO will continue to be a critical tool in extracting such insights.”
Reference: “The Image of the M87 Black Hole Reconstructed with PRIMO” by Lia Medeiros, Dimitrios Psaltis, Tod R. Lauer and Feryal Özel3, 13 April 2023, The Astrophysical Journal Letters.
DOI: 10.3847/2041-8213/acc32d
Development of the PRIMO algorithm was enabled through the support of the National Science Foundation Astronomy and Astrophysics Postdoctoral Fellowship.
“Amante de los viajes. Pionero de Twitter. Ávido gurú de la televisión. Aficionado a Internet galardonado”.